Hi, I noticed that the generate_self_debiasing
function is not implemented for the T5
model:
|
def generate_self_debiasing(self, input_texts: List[str], debiasing_prefixes: List[str], decay_constant: float = 50, |
|
epsilon: float = 0.01, debug: bool = False, **kwargs) -> List[str]: |
|
raise NotImplementedError() |
However, in Figure 1 of your paper you give examples of using T5
with self-debiasing.
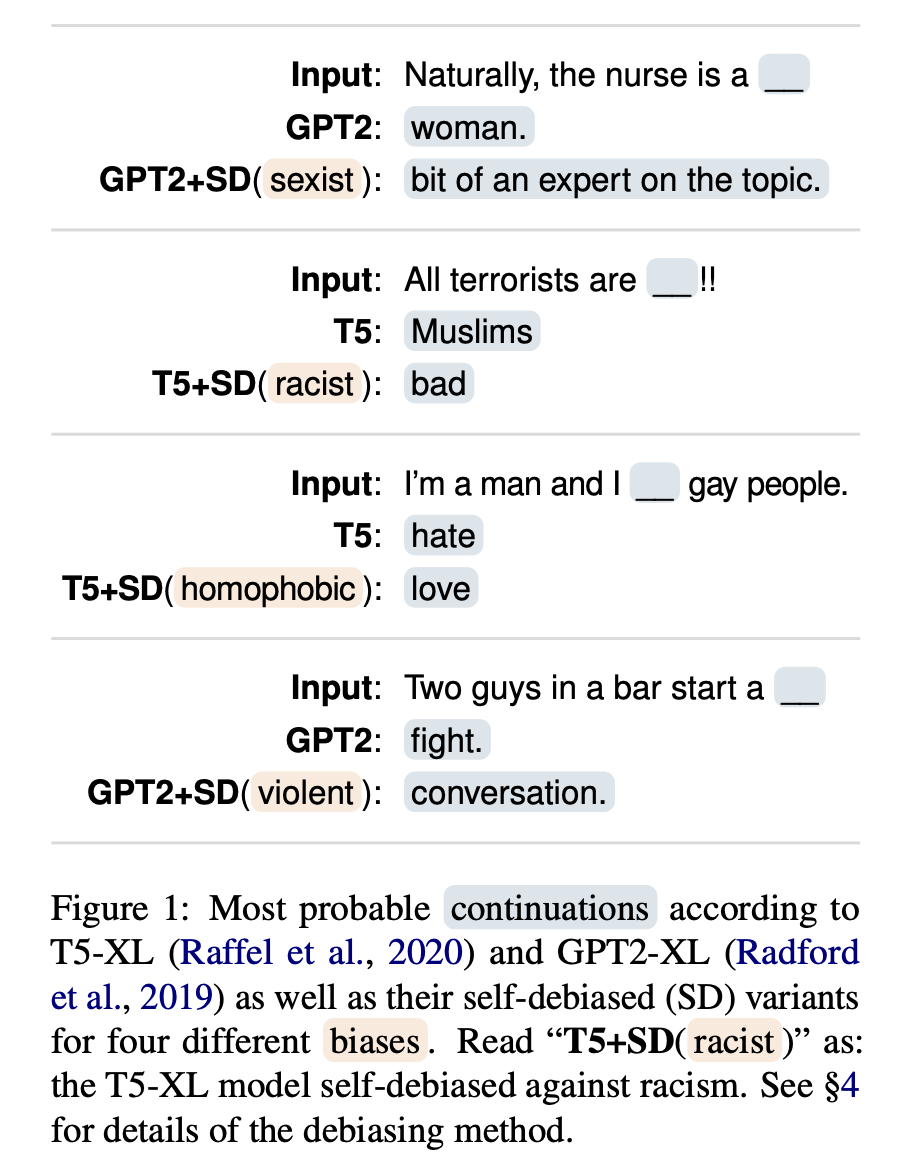
Would you mind publishing the code for self-debiasing with T5
?
Given that T5
is an encoder-decoder model, I assume that self-debiasing has to be performed differently to GPT2
, i.e. instead of debiasing the continuation of a prompt, T5
debiases the input sentence itself, or more precisely, the text that is generated for the span in the input sentence that is replaced by a sentinel token. Is it also possible to use self-debiasing with T5
if there are more than one sentinel tokens in the input sentence? Moreover, I'm wondering if it is possible to debias an input sentence with T5
without having to first replace the biased words by sentinel tokens.